Alpine regions are highly sensitive to the impacts of climate change, with snowmelt dynamics playing a crucial role in their hydrological processes. Accurate modeling of snowmelt processes is essential for predicting water availability and hazard assessment in these regions. Recently, the increasing availability of radar remote sensing products has offered great potential for improving our capability to understand and monitor snowmelt processes at high spatial and temporal resolutions.
The “SnowTinel” project aims to better understand the capability of radar remote sensing to detect, monitor, and predict snowmelt processes in mountain regions. This interdisciplinary project involves expertise in snow and hydrological process modeling, in situ snow characterization, and remote sensing monitoring. The project is a collaboration between the WSL Institute for Snow and Avalanche Research SLF (WSL/SLF) in Davos and EURAC Research in Bolzano, supported by the Swiss National Science Foundation and the Province of South Tyrol in Italy.
The project’s primary goal is to improve our understanding of radar interactions with wet snowpack evolution and then evaluate the value of this information to enhance snowmelt modeling at the catchment scale. The melting phase is usually not very accurately simulated in distributed snow models, leading to errors in the exact timing of discharge. Any additional measurement that could help reduce these inaccuracies is welcome.
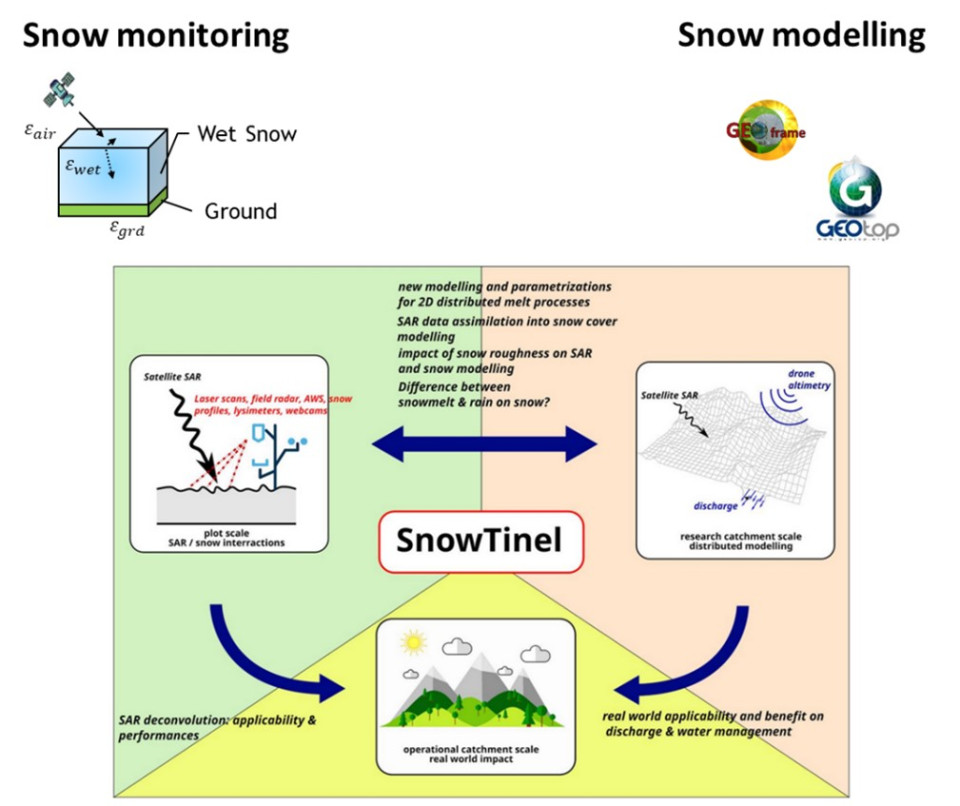
Figure 1: Conceptual scheme of the joint Swiss National Science Foundation – South Tyrol “Snowtinel” research project.
In this paper, we focus on the integration of remote sensing into a hydrological modeling framework to improve snowmelt prediction. A representative variable of snowmelt is the snow water equivalent (SWE). However, SWE measurements are rare and limited to point scales, making it difficult to obtain accurate spatialized estimates. Remote sensing products offer a unique opportunity to provide spatialized observations that can be exploited for SWE retrieval. Using optical remote sensing data from MODIS and Sentinel-2, radar SAR data from Sentinel-1, and in situ observations, Premier et al. (2023) developed a multi-source data method to reconstruct daily SWE at high spatial resolution (25 m). More specifically, accurate information about snow persistence, which is linked to the SWE amount, is retrieved from optical satellites, while accurate information about the runoff onset is retrieved from SAR data.
In this work, we investigate the effectiveness of this approach in estimating SWE for the alpine catchments of Dischma, a very well-monitored small catchment located near the city of Davos, Kanton Graubünden, Switzerland (~40 km²), and the Venosta catchment, a larger catchment located in the northwestern part of Südtirol, Italy (~1500 km²). Accurate snowmelt estimation is needed here as water is intensively used for various purposes, including hydropower and irrigation. We compare the results with those obtained using state-of-the-art hydrological models such as GEOframe (Formetta, 2013) or GEOtop (Rigon et al., 2006) and measurement data.
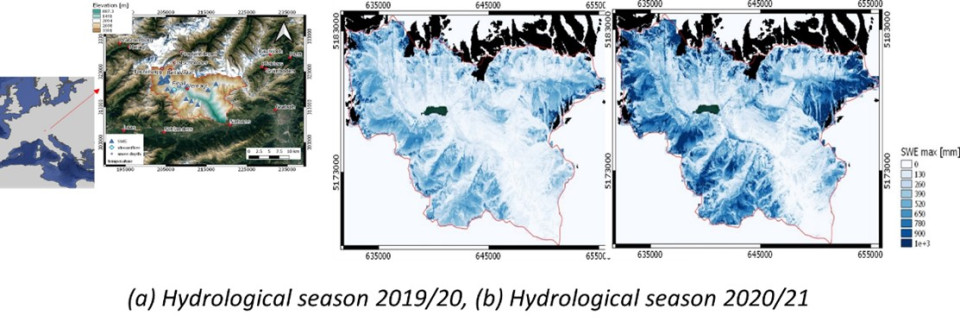
Figure 2: Example of high-resolution SWE estimation for the Senales are in the Venosta catchment in Italy.
Results show that, in terms of total spatial average, hydrological models tend to estimate SWE better than the satellite product of Premier et al. (2023), while the latter has a better capability to estimate spatial patterns at high resolution (~50 m). Moreover, Premier et al. (2023) does not consider glaciers and the presence of reservoirs. In this work, we will evaluate how to combine the Premier et al. (2023) product with GEOframe hydrological model results to substantially improve our ability to estimate snowmelt-related phenomena in alpine regions, especially for poorly monitored catchments.
Quiz question
What is the advantage of using the combination of high-resolution satellite data and hydrological models for the estimation of the snow water equivalent?
Have a product that can reproduce spatial patterns well and at the same time it is bound to a water mass balance.
Have a product that can reproduce spatial patterns well and at the same time it is bound to a water mass balance.
No advantages have been found using this method.